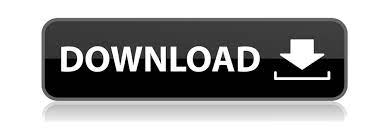
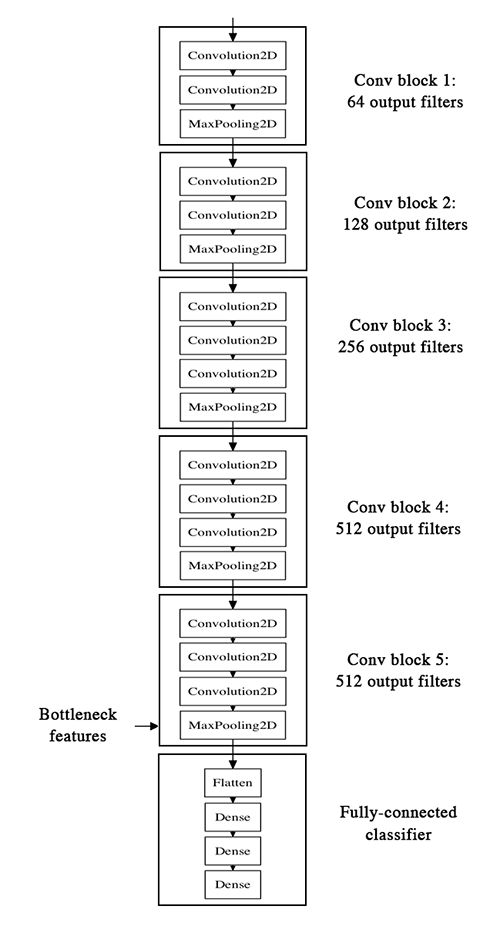
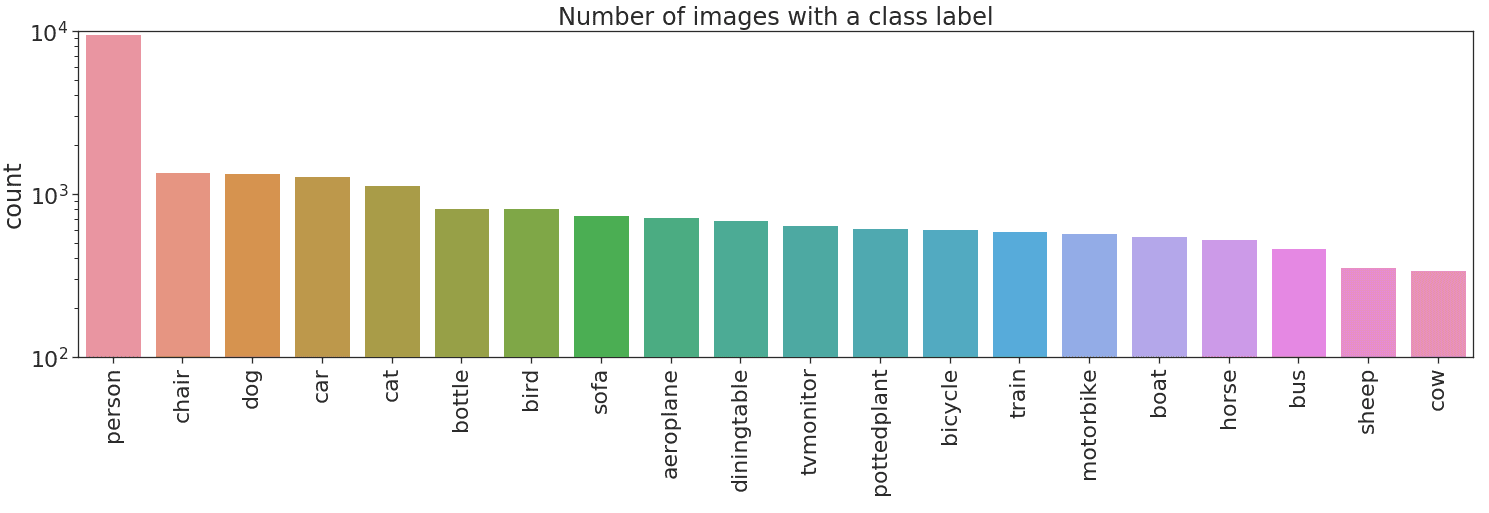
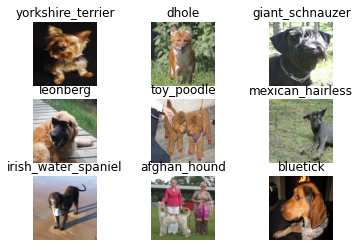
Still, you should keep in mind that you can augment the data for the ML problems as well.įor finer control you can write your own augmentation pipeline. That is why throughout this article we will mostly talk about performing Data Augmentation with various DL frameworks. In general, DA is frequently used when building a DL model. It means that Data Augmentation is also good for enhancing the model’s performance. However, we can improve the performance of the model by augmenting the data we already have. In general, having a large dataset is crucial for the performance of both ML and Deep Learning ( DL) models. Let’s make this clear, Data Augmentation is not only used to prevent overfitting. It is a good practice to use DA if you want to prevent overfitting, or the initial dataset is too small to train on, or even if you want to squeeze better performance from your model. Best practices, tips, and tricks What is Data Augmentation?ĭata Augmentation is a technique that can be used to artificially expand the size of a training set by creating modified data from the existing one.
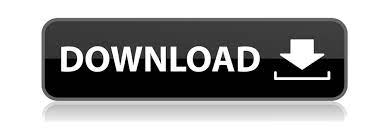